- Experimentation
An Ultimate Starter Guide to A/B Testing with Best Practices
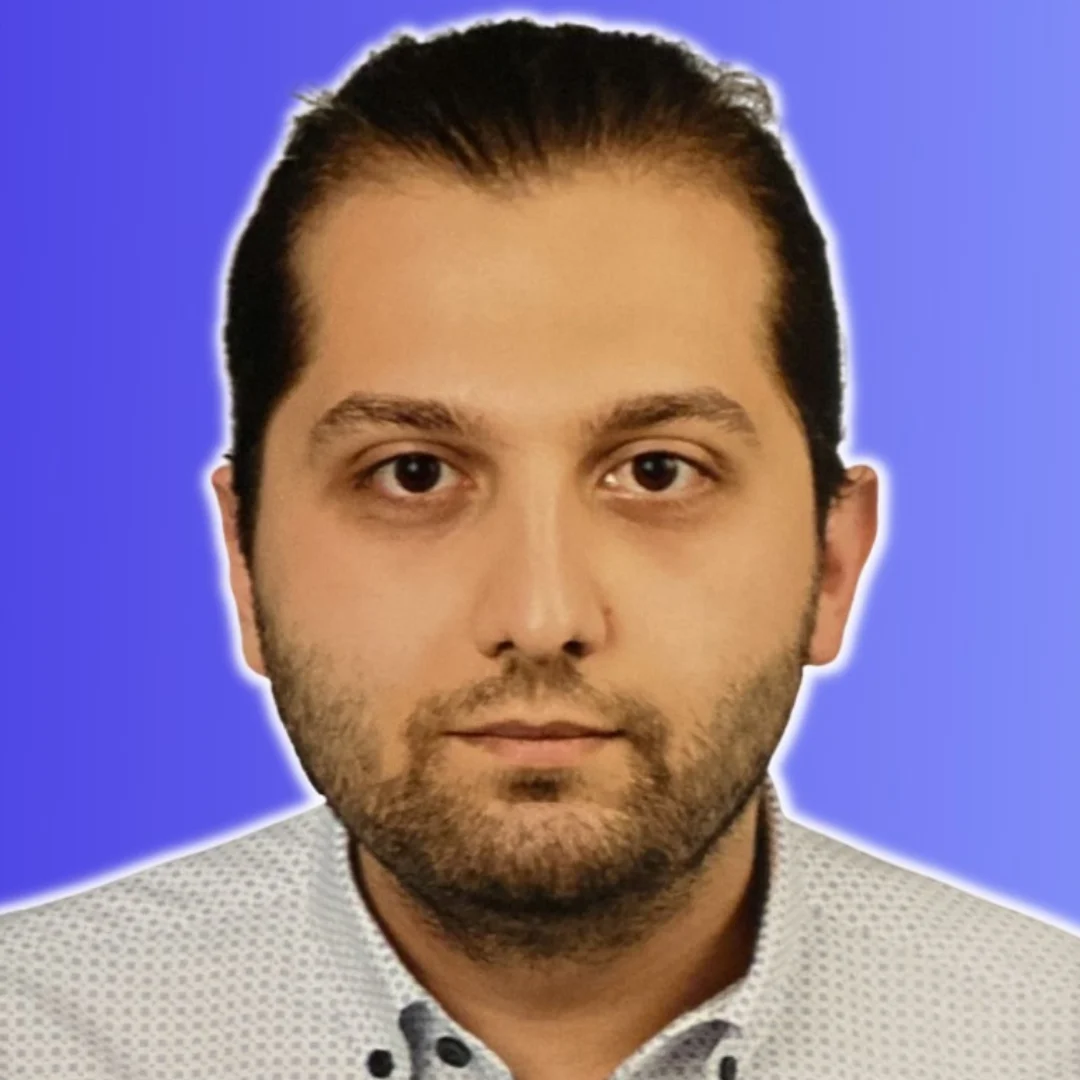
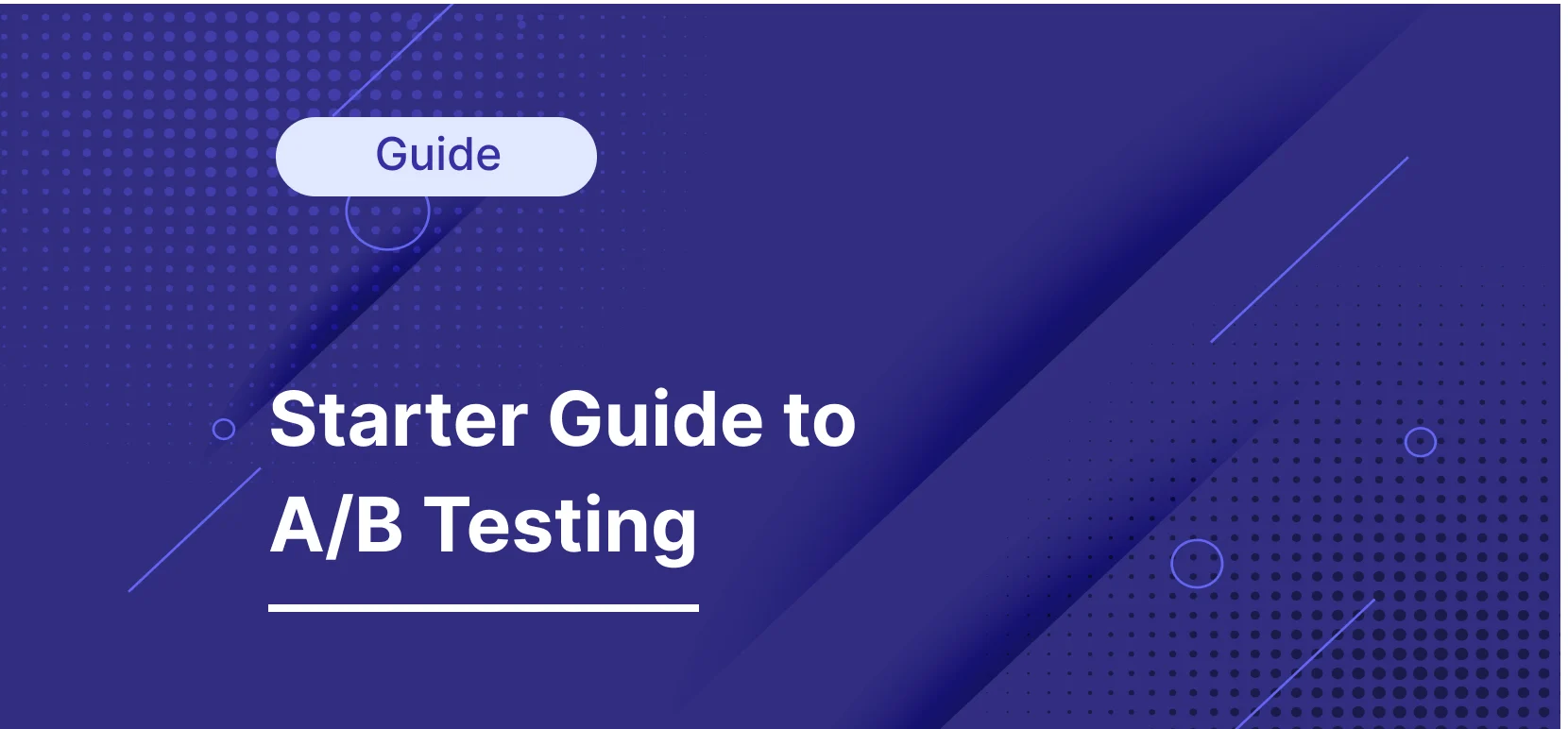
In the digital world, every click, every view, and even every scroll can provide valuable insights. But how do we make sense of all these interactions? How do we know what works and what doesn't?
The answer lies in A/B testing.
In the realm of marketing, it's easy to rely on our intuition when crafting landing pages, drafting emails, or designing call-to-action buttons. However, making decisions based purely on a "gut feeling" can often lead us astray. Instead, we need concrete data to guide our choices—this is where A/B testing shines.
But let's be honest, diving into A/B testing can feel like venturing into a jungle without a map if you're new to it. That's where this guide comes in. We've designed this ultimate starter guide to provide you with a roadmap to navigate the A/B testing landscape. Not only will we break down the basics, but we'll also share best practices to ensure your first foray into A/B testing is a resounding success.
What Is A/B Testing?
A/B testing is a method in which two versions of a webpage, email, or other piece of content are compared against each other to determine which one performs better. This is done by showing the two variants (let's call them A and B) to similar visitors at the same time.
The one that gives a better conversion rate, wins!
The conversion rate can be any desired action such as clicking on a button, signing up for a newsletter, making a purchase, or any other goal of the webpage.
A/B testing allows you to make more out of your existing traffic. While the cost of acquiring paid traffic can be huge, the cost of increasing your conversions is minimal. For example, if you have a bounce rate of 50%, getting 50% more traffic would cost a significant amount of money. But if you could reduce the bounce rate to just 25% with A/B testing, the increase in conversions would be equivalent to having increased your traffic by 50%, but for free!
In essence, A/B testing allows you to base your decisions on actual user behavior and data, rather than assumptions.
Why to Start with A/B Testing?
In the ever-evolving digital landscape, standing still is a surefire way to get left behind. To stay competitive and continuously improve, businesses must constantly test, learn, and adapt.
One of the most effective ways to do this is through A/B testing.
If you're still unsure whether to incorporate A/B testing into your strategy, here are three compelling reasons to start now:
Higher ROI from the Same Traffic
A/B testing is a cost-effective method of getting more value from your existing traffic.
By making small tweaks and observing the results, you can significantly increase conversions without needing to attract more visitors. This optimization of your current assets leads to a higher return on investment (ROI).
Data-Driven Decision Making
Guesswork and assumptions have no place in a successful digital strategy. A/B testing enables you to make decisions based on real, actionable data. This means you're not just shooting in the dark; every change is calculated and backed by evidence. In turn, this increases the likelihood of success and reduces the risk of costly mistakes.
Learn More About Your Audience
Last but certainly not least, A/B testing provides valuable insights into your audience's preferences and behavior.
By testing different elements, you can learn what resonates with your visitors and what doesn't. This understanding allows you to tailor your user experience to meet your audience's needs better, leading to increased satisfaction and loyalty.
To sum up, A/B testing is not just a nice-to-have—it's an essential tool for any business looking to optimize their digital presence, make informed decisions, and truly understand their audience.
What Are the Common A/B Testing Goals?
A/B testing is a valuable tool used by businesses to achieve specific goals. The most common goals of A/B testing include:
Higher Conversion Rate
One of the primary goals of A/B testing is to increase the conversion rate.
This could be anything from getting more people to sign up for a newsletter, increasing the number of downloads for a digital product, or boosting sales on an e-commerce website.
By testing different versions of a webpage, businesses can determine which elements (like headlines, images, or call-to-action buttons) are most effective at driving conversions.
Lower Bounce Rate
Bounce rate refers to the percentage of visitors who leave a website after viewing only one page.
A high bounce rate could indicate that your website's design, content, or usability is not appealing or intuitive for visitors.
Through A/B testing, you can experiment with different layouts, colors, content, and more to create a website that keeps visitors engaged and encourages them to explore more pages, thereby lowering the bounce rate.
Lower Cart Abandonment
Cart abandonment is a significant issue for e-commerce businesses. It happens when customers add products to their online shopping cart but leave the website without completing the purchase.
A/B testing can help identify and fix issues that might be causing cart abandonment. For instance, you could test different checkout processes, payment options, shipping costs, or return policies to see what leads to higher completion rates.
By setting clear, measurable goals, businesses can use A/B testing to make data-driven decisions and continuously improve their user experience, leading to more successful outcomes.
What to A/B Test on Websites?
A/B testing on websites can cover a wide variety of elements, each of which could potentially impact a user's experience and actions. Here are some key aspects you could consider for A/B testing:
Headline
Headlines are a crucial component of your website that can significantly impact user engagement and conversion rates. When A/B testing headlines on your website, consider experimenting with the following aspects:
Length: The length of your headline can influence its readability and the user's understanding. Some users might prefer shorter, more concise headlines, while others might respond better to longer ones. Test different lengths to find out what works best for your audience.
Tone of Voice: The tone of your headline can set the mood for the rest of your content. Experimenting with different tones, such as formal, casual, humorous, or urgent, can help you understand what resonates most with your audience.
Promise or Value Proposition: The promise in your headline tells your visitors what they stand to gain from your product, service, or content. Testing different promises can help you identify what your audience finds most appealing.
Specific Keywords: Including specific keywords in your headlines can improve SEO and attract more targeted traffic. Experiment with different keywords to see which have the most impact.
Remember, A/B testing is not just about finding what works, but understanding why it works. By testing these elements, you can gain valuable insights into your audience's preferences and behaviors, allowing you to optimize your headlines and overall website experience for better results.
CTA
CTAs are another crucial component of any website. When conducting A/B testing for the Call-to-Action (CTA) on your website, consider experimenting with these aspects:
Copy: The copy of your CTA is critical as it communicates what action you want your visitors to take. Experiment with different verbs or action phrases to see which drives more clicks.
Placement: The location of your CTA on the page can significantly impact its visibility and, consequently, its effectiveness. Test different placements (e.g., above the fold, at the end of the page, in a sidebar) to find the most optimal spot.
Size: The size of your CTA button can also affect its visibility and click-through rate. Larger buttons might be more noticeable, but they can also be overwhelming if not designed properly. Test different sizes to find a balance between visibility and aesthetics.
Design: The design elements of your CTA, such as color, shape, and use of whitespace, can greatly influence its attractiveness and clickability. Experiment with different design elements to make your CTA stand out and appeal to your audience.
Font: The font used in your CTA can impact its readability and perception. Test different fonts, font sizes, and text colors to ensure your CTA is easy to read and aligns with your brand image.
Remember, the goal of A/B testing is to find the combination of elements that maximizes engagement and conversions. By systematically testing these elements, you can optimize your CTAs and improve your website's overall performance.
Design, Layout, and Navigation
The design and layout of a website play a critical role in user engagement, conversion rates, and overall user experience. A/B testing these elements is vital as it provides empirical data on what works best for your audience. By experimenting with different designs and layouts, you can identify what encourages users to stay longer, interact more, and ultimately convert.
When conducting A/B testing for the design and layout of your website, consider experimenting with these aspects:
Navigation Structure: The navigation structure of your website can significantly affect user experience. Test different structures to see which is most intuitive and effective for your users.
Page Layout: The arrangement of elements on a page can impact how users interact with your content. Experiment with different layouts to find what works best.
Color Scheme: Colors can evoke different emotions and responses from users. Test different color schemes to see which is most appealing to your audience.
Typography: The style and size of your text can influence readability and user engagement. Test different fonts, font sizes, and line spacing to find what's most readable.
Images vs Text: Some users might respond better to visual content, while others prefer text. Experiment with the balance between images and text to see what your audience prefers.
Forms Design: If your site uses forms (for newsletter sign-ups, contact information, etc.), the design of these forms can impact conversion rates. Test different designs, form lengths, and form fields to optimize your forms.
Buttons: The design of your buttons (including CTAs) can influence click-through rates. Experiment with different colors, shapes, and sizes to find what's most effective.
Customer Spotlight
One of the tests Ace & Tate rolled out was for the primary navigation to improve engagement with topline categories and the underlying content to help them buy glasses.
They ran an A/B test where they showed either help or services as an entry point in the main navigation and measured CTR to the navigation and singular entry points.
The test resulted in an uplift of 54% on the desktop; however, the real win was on mobile, with an 87% increase in click rate. The streamlined content under the services item also saw improvements, with ~50% more users engaging with the links.
The result? New navigation was rolled out to all retail countries following the test.
Get in touch to learn how Ninetailed personalization, experimentation, and insights works inside your CMS
Price
A/B testing for price is a crucial strategy for optimizing business profits and customer satisfaction.
Different pricing structures, levels, and strategies can significantly impact consumer behavior and purchase decisions.
By using A/B testing, businesses can experiment with various price points, discounts, bundle options, and more to identify what maximizes revenue and conversions without deterring potential customers. It also helps in understanding customers' price sensitivity, which can inform future pricing decisions.
Thus, A/B testing for price is not just about increasing immediate sales; it's about gaining insights that can drive long-term business strategy and growth.
Customer Spotlight
The second example from Ace & Tate was a bit more complicated than the first one, as there was one more variable.
Ace & Tate ran an A/B/C test to validate a hypothesis that showing clearer pricing earlier in the funnel will give users more confidence to proceed with a purchase. The test ran with a variant for a from price and another with a fixed price.
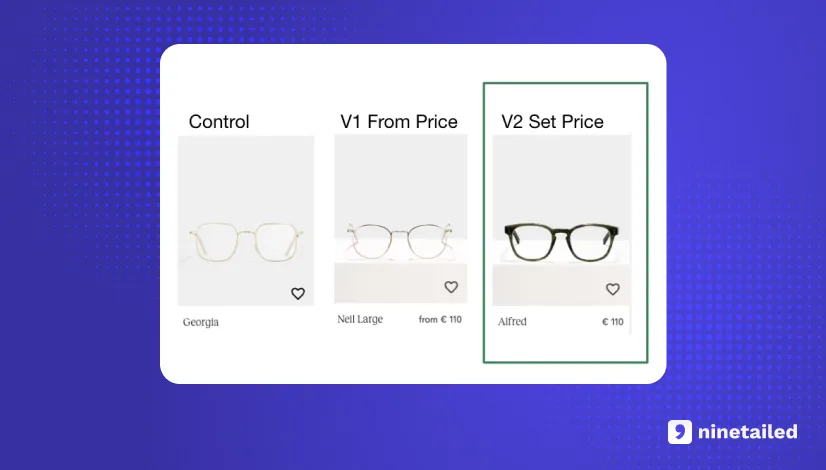
Although they expected higher CTR to product pages, there actually was a decline in users moving forward in the funnel. However, when Ace & Tate dug deeper into the full funnel, they saw that in both variants, the users that moved forward had a higher propensity to complete their product configuration, and in the case of the fixed price variant, they were also 8% more likely to convert to a sale, leading to higher purchases than the control group despite the decline earlier in the funnel – a great example that sometimes it pays to look past your initial success metrics to get the full picture.
More A/B Testing Ideas
What to A/B Test in Emails?
Subject Line
A/B testing in email marketing can significantly improve your open rates, click-through rates, and conversions. Here are a few elements to consider when A/B testing your email subject lines:
Numbers: Numbers stand out in text and can make your email subject line more eye-catching. Try testing subject lines with numbers against those without. For example, "5 Ways to Improve Your Customer Experience with Personalization" versus "How to Improve Your Customer Experience with Personalization".
Questions: Questions can spark curiosity and engage the reader's mind, making them more likely to open the email. Test a question-based subject line against a statement. For instance, "Want to Boost Your Conversions with Personalization?" versus "Boost Your Conversions with Personalization".
Emojis: Emojis can add personality and visual appeal to your subject lines, potentially increasing open rates. However, they might not be suitable for all audiences or brands. A/B testing can help you determine if emojis are effective for your specific audience. For example, "Generate More Revenue with Personalization 🌍" versus "Generate More Revenue with Personalization".
Design and Layout
A/B testing in email design and layout can help you understand what elements engage your audience the most, leading to higher click-through rates and conversions. Here are some aspects to consider:
Email Format: Test plain text emails against HTML emails. While HTML emails allow for more creativity and branding, plain text emails can sometimes feel more personal and less promotional, potentially leading to higher engagement.
Content Placement: The placement of your content can greatly impact how readers interact with your email. Test different layouts, such as single-column vs. multi-column, or changing the order of sections.
Images and Videos: Visuals can make your emails more engaging, but they can also distract from your message or lead to deliverability issues. Test emails with and without images or videos, or try different types and amounts of visuals.
Call-to-Action (CTA) Design: Your CTA is arguably the most important part of your email. Experiment with different button colors, sizes, shapes, and text to see what gets the most clicks.
Typography and Colors: The fonts and colors you use can impact readability and mood. Try different font sizes, styles, and color schemes to see what your audience prefers.
Personalization: Personalizing emails can increase relevance and engagement. Test different levels of personalization, such as using the recipient’s name, referencing their past behavior, or tailoring content to their interests.
CTAs
CTAs are another crucial component of any email. When conducting A/B testing for the Call-to-Action (CTA) on your emails, consider experimenting with these aspects:
Copy: The copy of your CTA is critical as it communicates what action you want your readers to take. Experiment with different verbs or action phrases to see which drives more clicks.
Placement: The location of your CTA on the email can significantly impact its visibility and, consequently, its effectiveness. Test different placements (e.g., above the fold, at the end of the page, etc.) to find the most optimal spot.
Size: The size of your CTA button can also affect its visibility and click-through rate. Larger buttons might be more noticeable, but they can also be overwhelming if not designed properly. Test different sizes to find a balance between visibility and aesthetics.
Design: The design elements of your CTA, such as color, shape, and use of whitespace, can greatly influence its attractiveness and clickability. Experiment with different design elements to make your CTA stand out and appeal to your audience.
Font: The font used in your CTA can impact its readability and perception. Test different fonts, font sizes, and text colors to ensure your CTA is easy to read and aligns with your brand image.
Remember, the goal of A/B testing is to find the combination of elements that maximizes engagement and conversions. By systematically testing these elements, you can optimize your CTAs and improve your email’s overall performance.
What Are the Statistical Approaches Used for A/B Testing?
Bayesian Approach
This approach is based on Bayes' theorem, a fundamental principle in probability theory and statistics that describes how to update the probabilities of hypotheses when given evidence. It's named after Thomas Bayes, who provided the first mathematical formulation of this theorem.
In the context of A/B testing, the Bayesian approach starts with a prior belief about the parameter we're interested in, such as the conversion rate for a webpage. As new data comes in from the A/B test, this prior belief is updated using Bayes' theorem to form a posterior belief. The posterior belief is a probability distribution that represents our updated knowledge about the parameter.
One of the key advantages of the Bayesian approach is that it provides a natural and intuitive way to understand the results of an A/B test. For instance, it can tell us the probability that version B is better than version A, given the data. This is a more direct and interpretable answer to the question we're usually interested in.
Another advantage is its flexibility. The Bayesian approach allows us to incorporate prior knowledge and uncertainty, handle different types of data and models, and update our results as new data comes in.
However, the Bayesian approach also has its challenges. Specifying a prior can be subjective, and the calculations can be complex, especially for more complicated models.
Frequentist Approach
The Frequentist approach, on the other hand, is the classical method of statistical inference that most people learn in introductory statistics classes. It treats the true parameter as a fixed but unknown quantity, and it estimates this parameter using data from a sample.
In A/B testing, the Frequentist approach often involves hypothesis testing. The null hypothesis typically states that there's no difference between version A and version B. The alternative hypothesis states that there is a difference. Based on the data, we calculate a test statistic and a p-value. If the p-value is less than a certain threshold (usually 0.05), we reject the null hypothesis and conclude that there's a statistically significant difference.
The Frequentist approach is widely used and understood, and it provides clear procedures for estimation and hypothesis testing. However, it can be less flexible and harder to interpret than the Bayesian approach. For instance, the p-value is a commonly misunderstood concept, and it doesn't directly answer the question of interest in an A/B test.
Bayesian Approach | Frequentist Approach | |
---|---|---|
Basis | Based on Bayes' theorem. Involves updating a prior belief with new data to form a posterior belief. | Based on the concept of hypothesis testing. Involves estimating a fixed but unknown parameter based on a sample. |
Interpretation of Results | Provides a probability distribution that represents our updated knowledge about the parameter. Can answer questions like "What is the probability that version B is better than version A?" | Provides a p-value that represents the probability of observing the data (or more extreme data) if the null hypothesis is true. |
Advantages | More intuitive and direct answers. Greater flexibility. Ability to incorporate prior knowledge and uncertainty. | Widely used and understood. Clear procedures for estimation and hypothesis testing. |
Challenges | Specifying a prior can be subjective. Calculations can be complex for more complicated models. | P-value is a commonly misunderstood concept. Doesn't directly answer the question of interest in an A/B test. |
What Are the Steps to Perform an A/B Test?
Research
The research phase in the A/B testing process is crucial as it lays the foundation for the entire test. It's here where you gather valuable insights that will guide your testing strategy. Here's a closer look at what this step entails:
Gathering Data About Your Current Performance: Before you can improve, you need to understand your current situation. This involves collecting data about your website or marketing asset's performance. You might look at metrics such as bounce rates, conversion rates, time spent on a page, and more. These metrics give you a baseline against which you can measure the impact of your A/B test.
Understanding Your Audience's Behavior: To create effective tests, you need to understand how your audience interacts with your website or marketing assets. This may involve using tools like heatmaps, session recordings, or user surveys to gain insights into your audience's behavior. For example, are there parts of your webpage that users tend to ignore? Are there steps in your checkout process where users often drop off? These insights can help you identify potential areas for testing.
Identifying Potential Areas for Improvement: Once you've collected data and gained a better understanding of your audience's behavior, you can start to identify potential areas for improvement. These could be elements on your webpage that are underperforming, steps in your user journey that are causing friction, or opportunities that you're currently not taking advantage of.
Studying Industry Trends: Finally, it's important to keep an eye on industry trends. What are your competitors doing? Are there new design trends, technologies, or strategies that could potentially improve your performance? By staying up-to-date with the latest developments in your industry, you can ensure that your A/B tests are not only optimizing your current performance but also helping you stay ahead of the competition.
In summary, the research phase in A/B testing is all about gathering as much information as possible to inform your testing strategy. It's about understanding where you are now, what's working and what's not, and identifying opportunities for improvement. This step is crucial for ensuring that your A/B tests are focused, relevant, and likely to drive meaningful improvements in your performance.
Formulate the Hypothesis and Identify the Goal
After conducting thorough research, the next phase in the A/B testing process is to formulate a hypothesis and identify the goal of your test. This step is vital as it sets the direction for your test and defines what success looks like. Here's an in-depth view of this process:
Formulating the Hypothesis: A hypothesis is a predictive statement that clearly expresses what you expect to happen during your A/B test. It's based on the insights gained during the research phase and should link a specific change (the variable in your test) to a predicted outcome. For example, "Changing the color of the 'Add to cart' button from blue to red will increase click-through rates." The hypothesis should be clear and specific, and it should be testable - that is, it should propose an outcome that can be supported or refuted by data.
Formulating a strong hypothesis is crucial because it ensures that your A/B test is focused and has a clear purpose. It also provides a benchmark against which you can measure the results of your test.
Identifying the Goal: Alongside the hypothesis, you need to clearly define the goal of your A/B test. The goal is the metric that you're aiming to improve with your test, and it should be directly related to the predicted outcome in your hypothesis. Common goals in A/B testing include increasing conversion rates, improving click-through rates, reducing bounce rates, boosting sales, or enhancing user engagement.
Identifying the goal is important as it gives you a clear measure of success for your test. It ensures that you're not just making changes for the sake of it, but that you're working towards a specific, measurable improvement in performance.
Design the Experiment
The design phase of an A/B test is where you plan out the specifics of your test, including the elements to be tested, the sample size, and the timeframe. This is a crucial phase as it sets the groundwork for the execution of your test. Here's a detailed look at what this step involves:
Test the Right Elements That Serve Your Business Goals
One of the most important decisions you'll make during the design phase is choosing which elements to test. These elements could be anything from headlines, body text, images, call-to-action buttons, page layouts, or even entire workflows. The key here is to choose elements that have a direct impact on your business goals. For instance, if your goal is to increase conversions, you might choose to test elements that directly influence the conversion process, such as the call-to-action button or the checkout process.
It's also important to test only one element at a time (or a group of changes that constitute one distinct variant) to ensure that you can accurately attribute any changes in performance to the element you're testing.
Determine the Correct Sample Size
Another key decision during the design phase is determining the sample size for your test. The sample size refers to the number of users who will participate in your test. The correct sample size is crucial for ensuring that your test results are statistically significant, i.e., not due to chance.
Factors that can influence the appropriate sample size include your website traffic, conversion rates, and the minimum effect you want to detect. Online sample size calculators can help you determine the right sample size for your test.
Remember, a small sample size might lead to inaccurate results, while an excessively large one could waste resources.
Decide on Timeframe
Lastly, you need to decide how long your test will run. The duration of your test should be long enough to capture a sufficient amount of data but not so long that external factors (like seasonal trends) could influence the results. A typical timeframe for an A/B test is at least two weeks, but this can vary depending on your website traffic and the nature of your business. For example, businesses with high daily traffic might achieve statistically significant results faster than those with lower traffic.
It's also crucial to run the test through complete business cycles to account for daily and weekly variations in user behavior.
Create Variants
Creating variants is a vital step in the A/B testing process. It involves making different versions of your webpage or marketing asset that you intend to test against each other. The purpose of this step is to see which version performs better in achieving your specified goal. Here's a closer look at what this step entails:
Creating Different Versions: In the context of A/B testing, a variant refers to a version of your webpage or marketing asset that has been modified based on your hypothesis. For instance, if your hypothesis is "Changing the color of the 'Add to cart' button from blue to red will increase conversions," you would create one variant of your webpage where the only change made is the color of the 'Add to cart' button.
This variant will then be tested against the original version (often referred to as the 'control') to see if the change leads to an increase in conversions. If you're testing more than one change, you would create multiple variants, each incorporating a different proposed change.
Ensuring Minimal Changes Between Variants: It's crucial that the changes between your control and the variants are minimal and isolated. This means that you should only test one change at a time. The reason for this is so you can accurately attribute any differences in performance to the specific element you're testing.
If you were to change multiple elements at once - say, the color of the 'Add to cart' button and the headline text - and saw an increase in conversions, you wouldn't be able to definitively say which change led to the improvement. By keeping changes minimal and isolated, you can ensure that your test results are accurate and meaningful.
Run the Test
Running the test is a key phase in the A/B testing process. This step involves using an A/B testing tool to present the different versions of your webpage or marketing asset (the 'control' and the 'variants') to your audience, and then collecting data on user interaction. Here's a more detailed look at what this step involves:
Serving Different Versions Randomly: One of the fundamental aspects of A/B testing is that the different versions of your webpage or marketing asset are served randomly to your audience. This means that when a visitor lands on your webpage, an A/B testing tool decides (based on a random algorithm) whether they see the control or one of the variants.
Randomization is essential to ensure that there's no bias in who sees which version, which could otherwise skew the results. For instance, if visitors from a certain geographical area were more likely to see one version than another, this could influence the results. Randomization ensures that all visitors have an equal chance of seeing any version, making the test fair and the results reliable.
Collecting Data on User Interaction: While the A/B test is running, the testing tool collects data on how users interact with each version. This may include metrics such as the number of clicks, time spent on the page, conversions, bounce rate, and so on, depending on your testing goal.
This data is crucial as it allows you to compare the performance of the control and the variants. By analyzing this data, you can determine which version led to better user engagement, higher conversion rates, or whatever your goal may be.
Analyze and Implement the Results
The final step in the A/B testing process is to analyze and implement the results. This phase involves interpreting the data collected during the test, evaluating the statistical significance, comparing the performance of the variants, and deciding on the next steps.
Measure the Significance
The first part of analyzing your A/B test results is measuring their statistical significance. This is done using a statistical significance calculator, which helps determine whether the differences observed between your control and variant(s) are due to the changes you made or just random chance.
In A/B testing, a result is generally considered statistically significant if the p-value (the probability that the observed difference could have occurred by chance) is less than 0.05. This means there's less than a 5% chance that the observed difference is due to randomness, making it reasonable to attribute the difference to the changes made in the variant.
Check Your Goal Metric and Compare
Once you've established that your results are statistically significant, the next step is to check your goal metric for each variant. This could be conversion rate, click-through rate, time spent on page, bounce rate, etc., depending on what your original goal was.
You'll need to compare these metrics for your control and variant(s) to see which version performed better. For example, if your goal was to increase conversions, you'd compare the conversion rates of your control and variant(s) to see which led to a higher conversion rate.
Take Action Based on Your Results
After analyzing and comparing your results, the final step is to take action. If one variant clearly outperformed the others, you would typically implement that change on your website or marketing asset.
However, if there's no clear winner, or if the results are not statistically significant, you would use the insights gained from the test to refine your hypothesis and design a new test. This could involve tweaking the changes made in the variant, testing a completely different element, or adjusting your sample size or test duration.
Start Planning the Next Test
A/B testing is not a one-time event but an ongoing process of learning, improving, and optimizing your website or marketing assets. Once you've analyzed and implemented the results of one test, the next step is to start planning the next one. Here's a more detailed look at this step:
Continuous Learning and Optimization: A/B testing is a cyclical process that involves constantly testing new hypotheses and implementing changes based on the results. It's a tool for continuous learning and optimization. Each test gives you more insights into your audience's behavior and preferences, which you can use to make data-driven decisions and improve your website or marketing asset's performance.
For example, if an A/B test shows that changing the color of your 'Add to cart' button from blue to red increases conversions, you might then test different shades of red to see which one performs best.
Looking for New Opportunities: After completing an A/B test, it's essential to reflect on the results and use them to identify new opportunities for testing. This could involve testing a different element of your webpage (like the headline, images, or layout), trying out a different change (such as changing the text of your call-to-action), or even testing a completely different hypothesis.
The goal is always to learn more about your audience and find ways to improve your results. For instance, if your first test didn't yield a clear winner, you might use the insights gained to refine your hypothesis and design a new test.
Implementing a Culture of Testing: The most successful companies have a culture of testing, where every decision is backed by data and every assumption is tested. By continuously planning and running new A/B tests, you can foster this culture in your own organization and ensure that your decisions are always data-driven.
Common Mistakes to Avoid While A/B Testing
A/B testing is an effective tool for optimizing user experience and driving engagement.
But, if done incorrectly, it can lead to costly mistakes and inaccurate results.
In the following blog post, we’ll cover 6 common A/B testing mistakes that are guaranteed to fail. You’ll learn and discover:
why it’s important to have a plan and set goals before you start testing,
how to create a control group, and
why you should avoid making too many changes at once.
By understanding the mistakes to avoid, you can save time, money, and resources. Moreover, you can ensure that your A/B testing efforts yield reliable and actionable results.
Read more about: 6 Common A/B Testing Practices That Will Certainly Fail
What Are the Main Challenges of A/B Testing?
Deciding What to Test
One of the first challenges in A/B testing is deciding what to test.
There are countless elements on a webpage that you could potentially test, from headlines and body text to images, buttons, layout, colors, and more. Determining which elements to focus on requires a solid understanding of your audience, your goals, and how different elements might impact user behavior.
Formulating Hypotheses
Creating a valid and effective hypothesis for your A/B test is not always straightforward. It requires a deep understanding of your users, your product, and your market.
Additionally, your hypothesis needs to be specific and measurable, and it should be based on data and insights rather than mere assumptions.
Locking In on Sample Size
Determining the right sample size for your A/B test can be tricky. If your sample size is too small, you might not get statistically significant results. If it's too large, you might waste resources on unnecessary testing.
You'll need to consider factors like your baseline conversion rate, the minimum detectable effect, and your desired statistical power and significance level.
Analyzing Test Results
Analyzing the results of an A/B test is more complex than just looking at which variant had a higher conversion rate. You need to calculate statistical significance to ensure that your results are not due to random chance.
Moreover, you should also consider other metrics and factors that might influence the results, such as seasonality or changes in user behavior over time.
Read more about: Which Personalization and Experimentation KPIs to Measure to Win
Maintaining a Testing Culture/Velocity
A/B testing is not a one-off activity but a continuous process of learning and optimization.
Maintaining a culture of testing and a high testing velocity can be a challenge, especially in larger organizations. It requires commitment from all levels of the organization, clear communication, and efficient processes for designing, implementing, and analyzing tests.
Read more about: Companies Are Failing in Personalization and Experimentation Because of Not Having a Testing Rhythm
Potential Effects on SEO
If not done correctly, A/B testing can potentially have negative effects on SEO.
For example, if search engines perceive your A/B test as an attempt to present different content to users and search engines (a practice known as "cloaking"), they might penalize your site. To mitigate this risk, you should follow best practices for A/B testing and SEO, such as using the rel="canonical" link attribute and the "noindex" meta tag.
The Relationship Between A/B Testing and Personalization
A/B testing and personalization are two powerful tools in the digital marketer's toolbox, and they are closely related. Both are aimed at optimizing the user experience and increasing conversions, but they do so in different ways.
A/B testing is a method of comparing two or more versions of a webpage or other marketing asset to see which one performs better. It involves showing the different versions to different segments of your audience at random, and then using statistical analysis to determine which version leads to better performance on a specific goal (such as click-through rate or conversion rate).
On the other hand, personalization is about tailoring the user experience based on individual user characteristics or behavior. This could involve showing personalized content or recommendations, customizing the layout or design of the site, or even delivering personalized emails or notifications.
So how do A/B testing and personalization relate to each other?
Firstly, A/B testing can be used to optimize your personalization strategies. For example, you might have a hypothesis that a certain type of personalized content will lead to higher engagement. You can use A/B testing to test this hypothesis - by creating two versions of a page (one with the personalized content and one without) and seeing which one performs better.
Conversely, personalization can also enhance the effectiveness of your A/B tests. By segmenting your users based on their characteristics or behavior, you can run more targeted and relevant A/B tests. This can lead to more accurate results and more effective optimizations.
In summary, while A/B testing and personalization are different techniques, they complement each other well. By combining the iterative, data-driven approach of A/B testing with the targeted, user-centric approach of personalization, marketers can create more effective and engaging user experiences.